Pengfei Ren, Yuanyuan Gao, Haifeng Sun, Qi Qi, Jingyu Wang, Jianxin Liao
State Key Laboratory of Networking and Switching Technology, Beijing University of Posts and Telecommunications
Abstract
Category-agnostic pose estimation (CAPE) aims to predict the pose of a query image based on few support images with pose annotations. Existing methods achieve the localization of arbitrary keypoints through similarity matching between support keypoint features and query image features. However, these methods primarily focus on mining information from the query images, neglecting the fact that support samples with keypoint annotations contain rich category-specific fine-grained semantic information and prior structural information. In this paper, we propose a Support-based Dynamic Perception Network (SDPNet) for the robust and accurate CAPE. On the one hand, SDPNet models complex dependencies between support keypoints, constructing category-specific prior structur
Overview
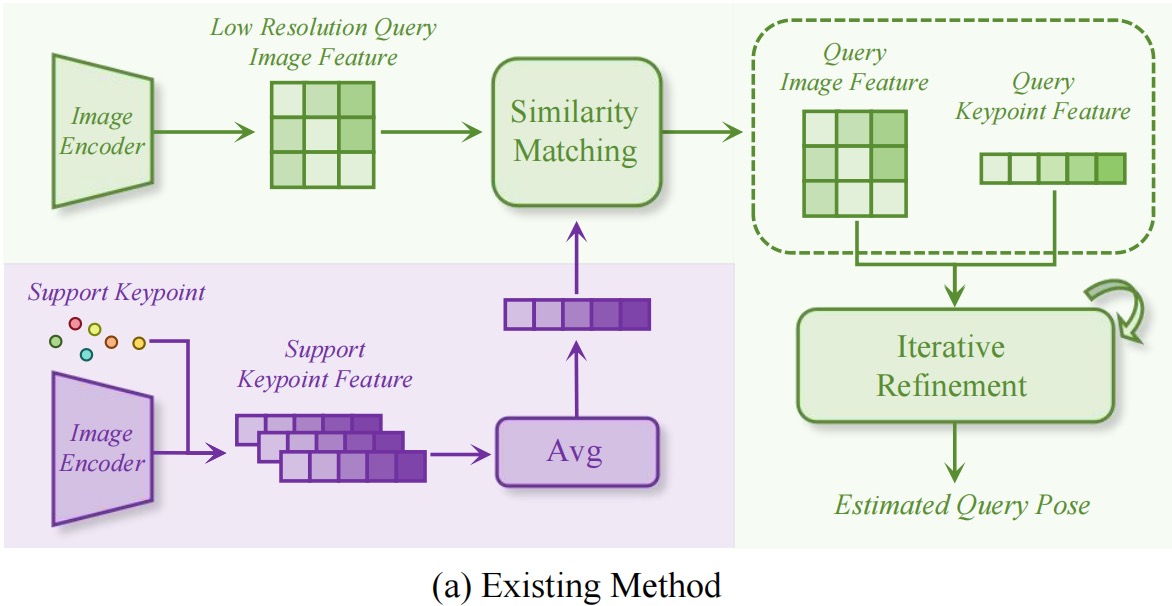
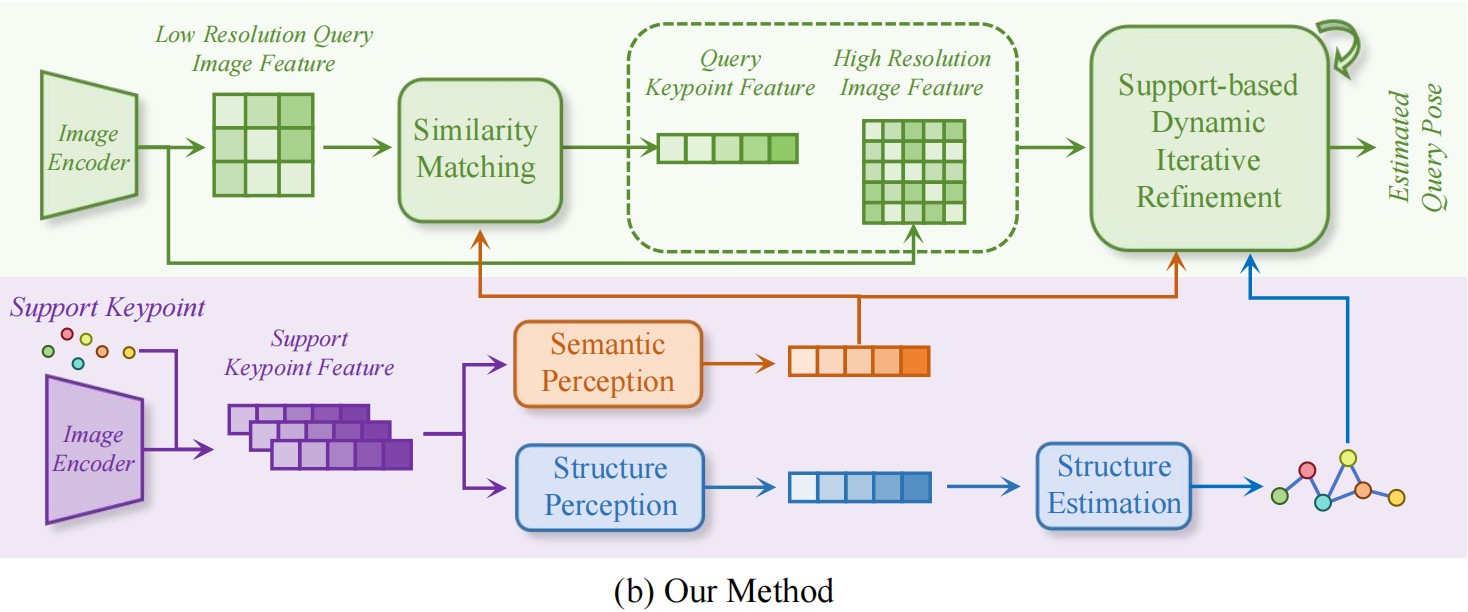
Comparison with existing methods. Previous methods mainly focus on information mining of query samples. Our method fully explores the support information, allowing support features to deeply participate in the iterative refinement.
Qualitative results

The bones are not the results predicted by our network, but provided by the dataset.
Bibtex
@inproceedings{ren2024dynamic,
title={Dynamic Support Information Mining for Category-Agnostic Pose Estimation},
author={Ren, Pengfei and Gao, Yuanyuan and Sun, Haifeng and Qi, Qi and Wang, Jingyu and Liao, Jianxin},
booktitle={Proceedings of the IEEE/CVF Conference on Computer Vision and Pattern Recognition},
pages={1921--1930},
year={2024}
}