Pengfei Ren, Haifeng Sun, Jiachang Hao, Jingyu Wang, Qi Qi, Jianxin Liao
Abstract
In this work, we study the cross-view information fusion problem in the task of self-supervised 3D hand pose estimation from the depth image. Previous methods usually adopt a hand-crafted rule to generate pseudo labels from multi-view estimations in order to supervise the network training in each view. However, these methods ignore the rich semantic information in each view and ignore the complex dependencies between different regions of different views. To solve these problems, we propose a cross-view fusion network to fully exploit and adaptively aggregate multi-view information. We encode diverse semantic information in each view into multiple compact nodes. Then, we introduce the graph convolution to model the complex dependencies between nodes and perform cross-view information interaction. Based on the cross-view fusion network, we propose a strong self-supervised framework for 3D hand pose and hand mesh estimation. Furthermore, we propose a pseudo multi-view training strategy to extend our framework to a more general scenario in which only single-view training data is used. Results on NYU dataset demonstrate that our method outperforms the previous self-supervised methods by 17.5% and 30.3% in multi-view and single-view scenarios. Meanwhile, our framework achieves comparable results to several strongly supervised methods.
Overview
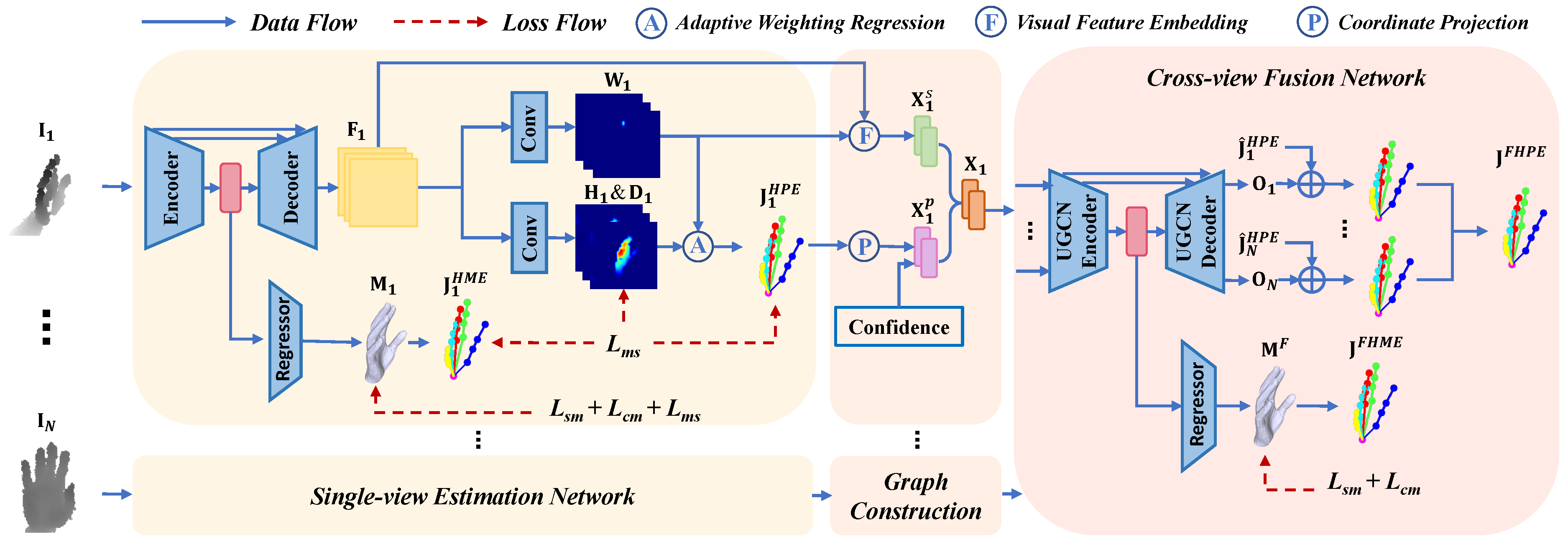
1️⃣ Pre-training SEN on Single-view Data: First, we use synthetic data for pre-training.
2️⃣ Training CFN to Improve Multi-view Fusion: Then, the two networks adopt a single-view model-fitting loss Lsm and a cross-view model-fitting loss Lcm to perform self-supervised learning on unlabeled real data.
3️⃣ Self-distillation to Improve SEN: Meanwhile, the single-view estimation network is trained to produce results consistent with the cross-view fusion network, which is called multi-view self-distillation loss Lms.
4️⃣ During the inference, only the single-view estimation network is utilized.
Qualitative Results
On ICVL and MSRA datasets, our method predicts more accurate 3D hand pose than ground-truth (GT).
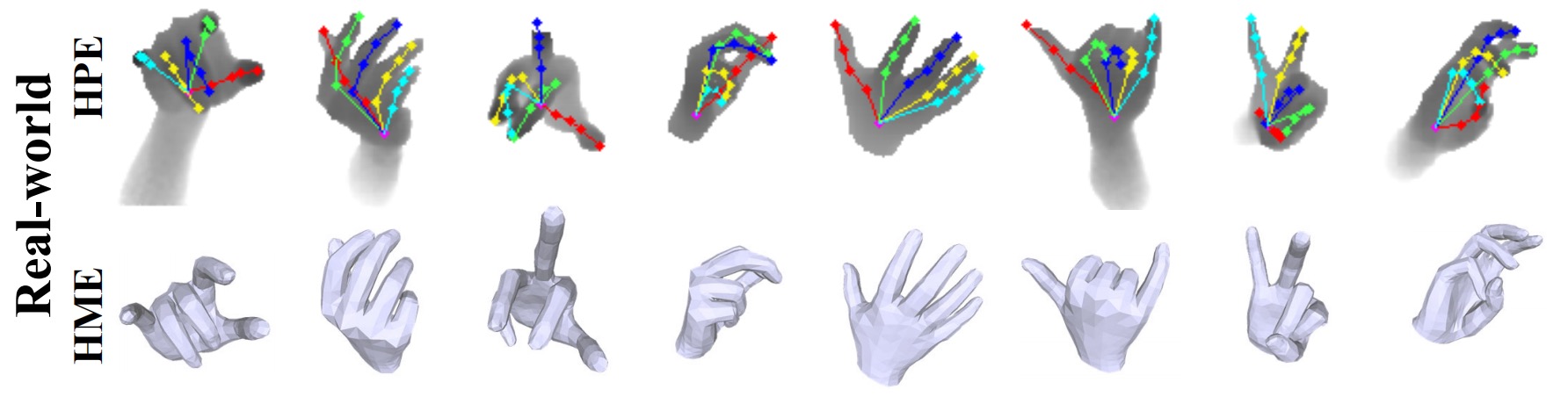
Comparison with SOTA:
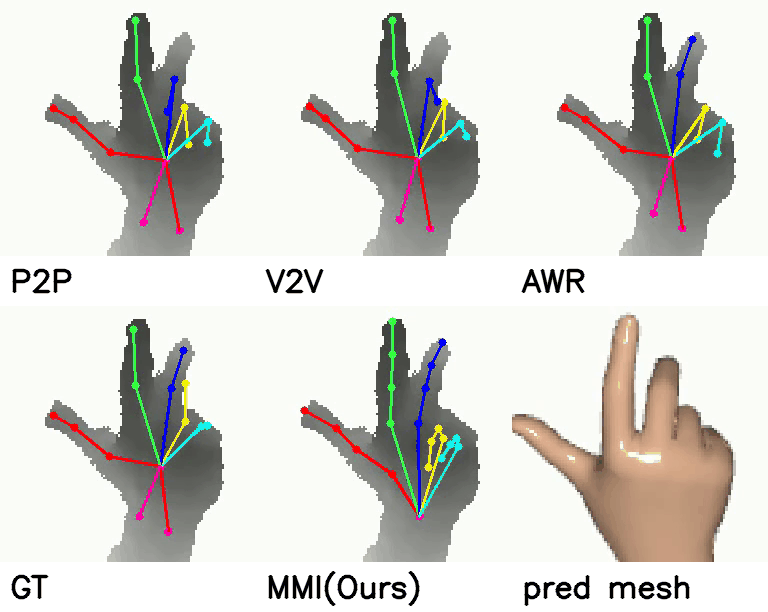
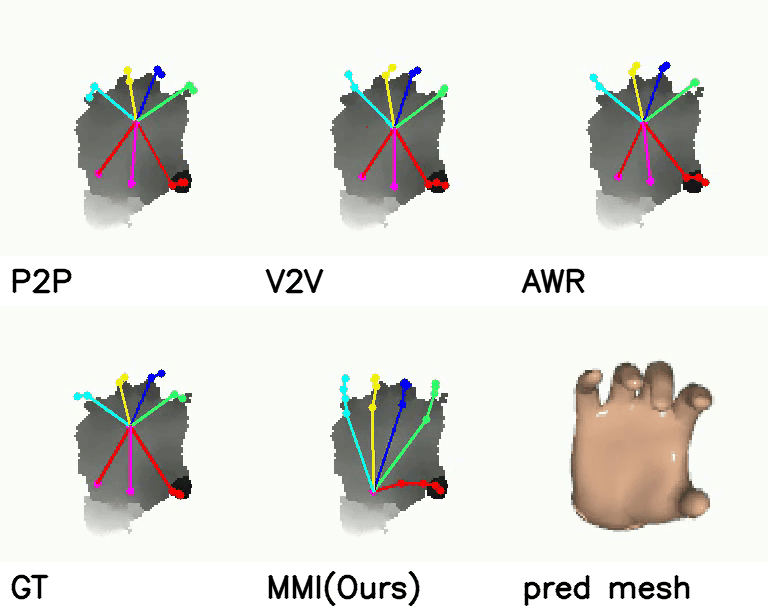
Comparison with SOTA
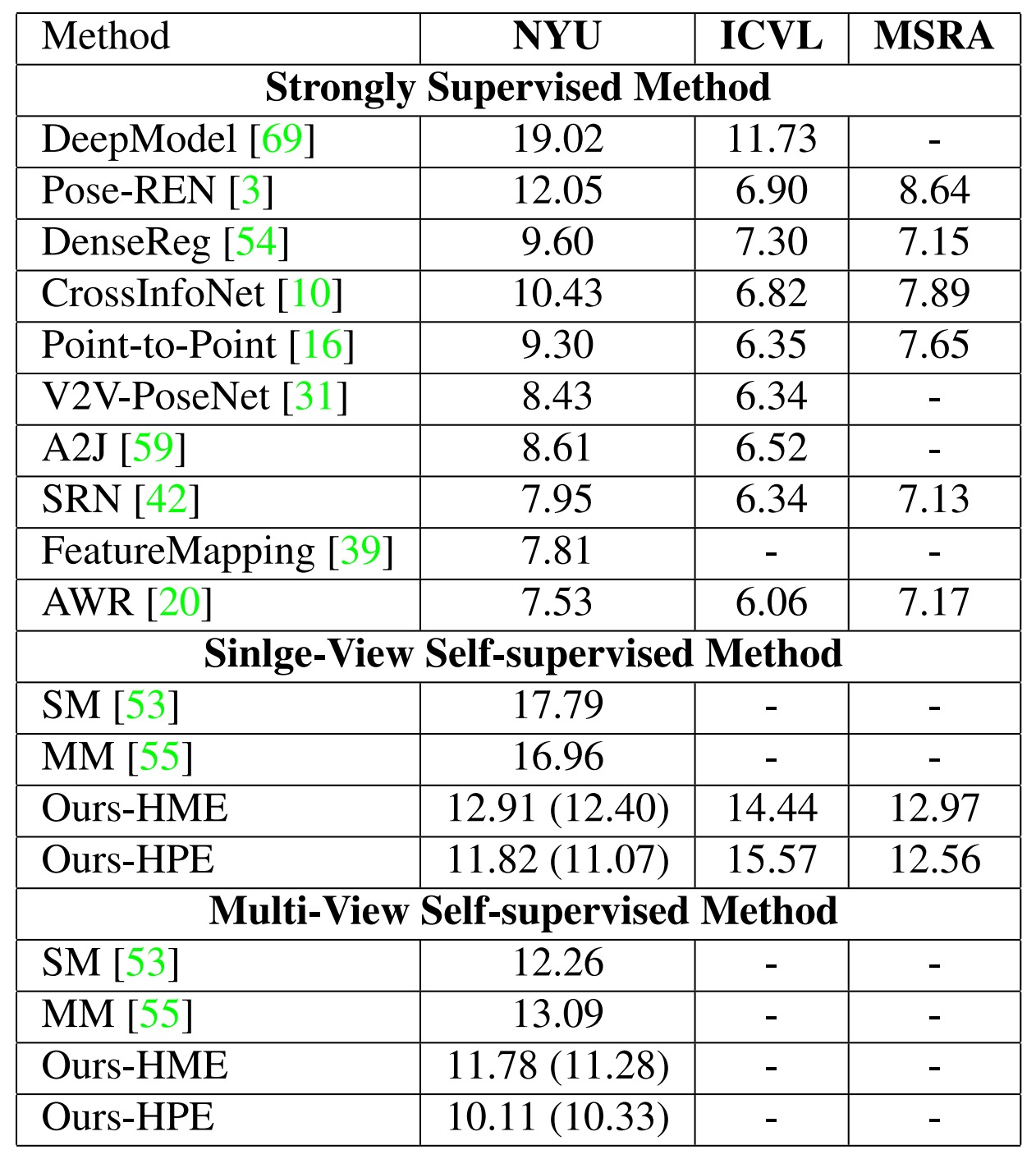
On NYU dataset, our method reduces the mean joint error by 17.5% (10.11 mm vs 12.26 mm) and 30.3% (16.96 mm vs 11.82 mm) in multi-view and single-view scenarios compared with SOTA self-supervised methods. Meanwhile, when comparing with strongly supervised methods, our model can get comparable performance.
Conclusion and Limitations
✅ First, we propose a cross-view fusion network to fully mine multi-view information, which provides accurate and robust guiding information for the single-view estimation network during self-supervised training.
✅ Then, by adopting a pseudo multi-view training strategy, we extend our framework to the single-view scenario.
✅ On the NYU dataset, our method outperforms the previous self-supervised methods by a large margin in both single-view and multi-view scenarios. On the ICVL and MSRA datasets, our method generates more accurate poses than annotations.
❌ Limitations: In the multi-view scenario, our method must use the camera extrinsic parameters, which is unfriendly to the scene where the camera position is constantly changing.
Bibtex
@inproceedings{ren2022mining,
title={Mining multi-view information: a strong self-supervised framework for depth-based 3D hand pose and mesh estimation},
author={Ren, Pengfei and Sun, Haifeng and Hao, Jiachang and Wang, Jingyu and Qi, Qi and Liao, Jianxin},
booktitle={Proceedings of the IEEE/CVF conference on computer vision and pattern recognition},
pages={20555--20565},
year={2022}
}