Pengfei Ren, Haifeng Sun, Jiachang Hao, Qi Qi, Jingyu Wang, Jianxin Liao
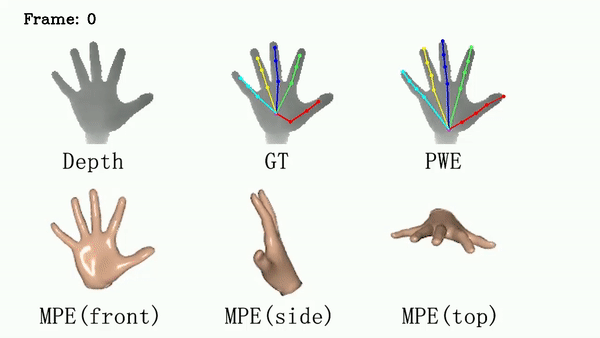
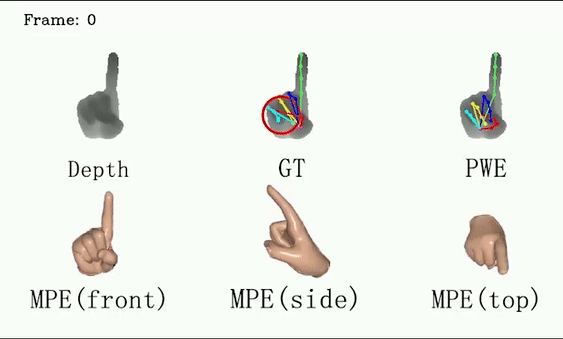
For ICVL and MSRA, we show that DSF is able to generate more reasonable hand poses than annotations.
We use red circles to locate errors in annotations.
Overview
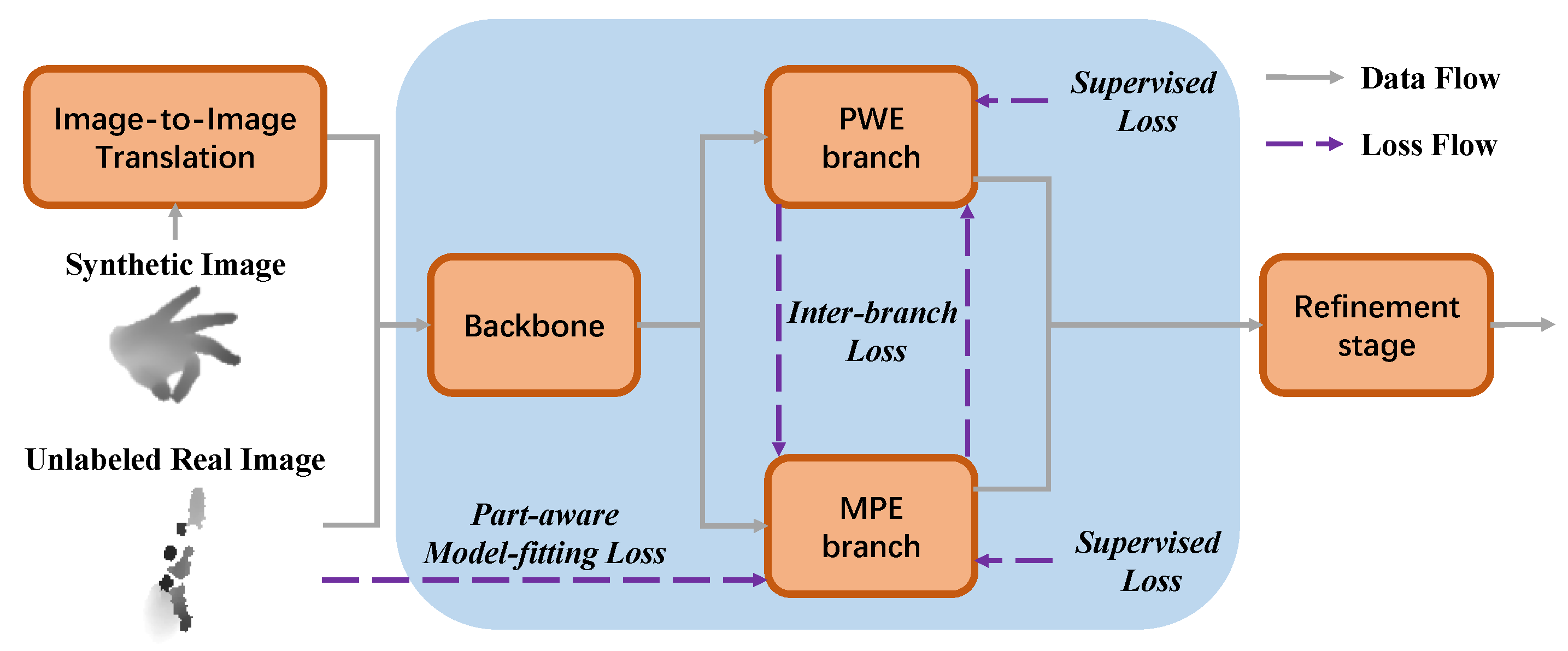
Through image-to-image translation technology, our framework can make better use of synthetic data for pre-training. The dual-branch design allows our framework to adopt a part-aware model-fitting loss for self-supervised learning on unlabeled real data while achieving high-accuracy pose estimation and flexible and robust hand model estimation simultaneously.
Qualitative Results
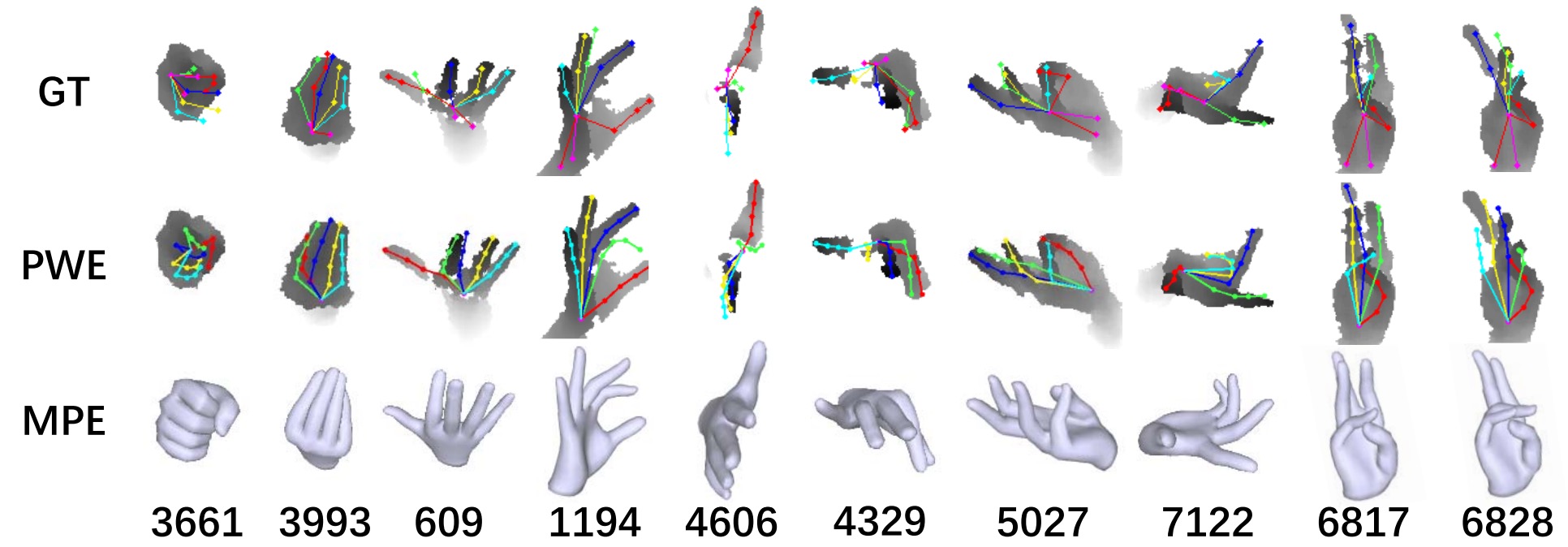
✅ DSF can maintain the rationality of hand pose when fingers contact intensively, such as clenching (3361) or when fingers are pressed together (3993).
✅ DSF is robust to extreme perspective (609, 4606) and the depth holes (1194, 4329). Although DSF’s predictions in missing regions do not completely consistent with the ground truth, they remain structurally reasonable.
✅ DSF can predict complex hand poses (5027, 7122, 6817) with high accuracy.
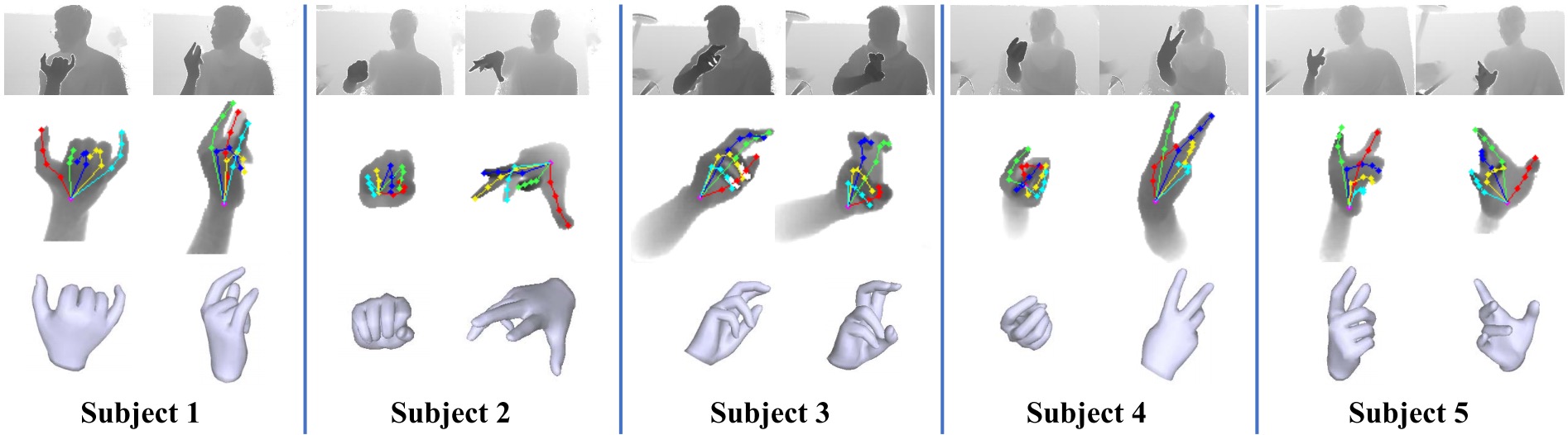
Comparison to SOTA
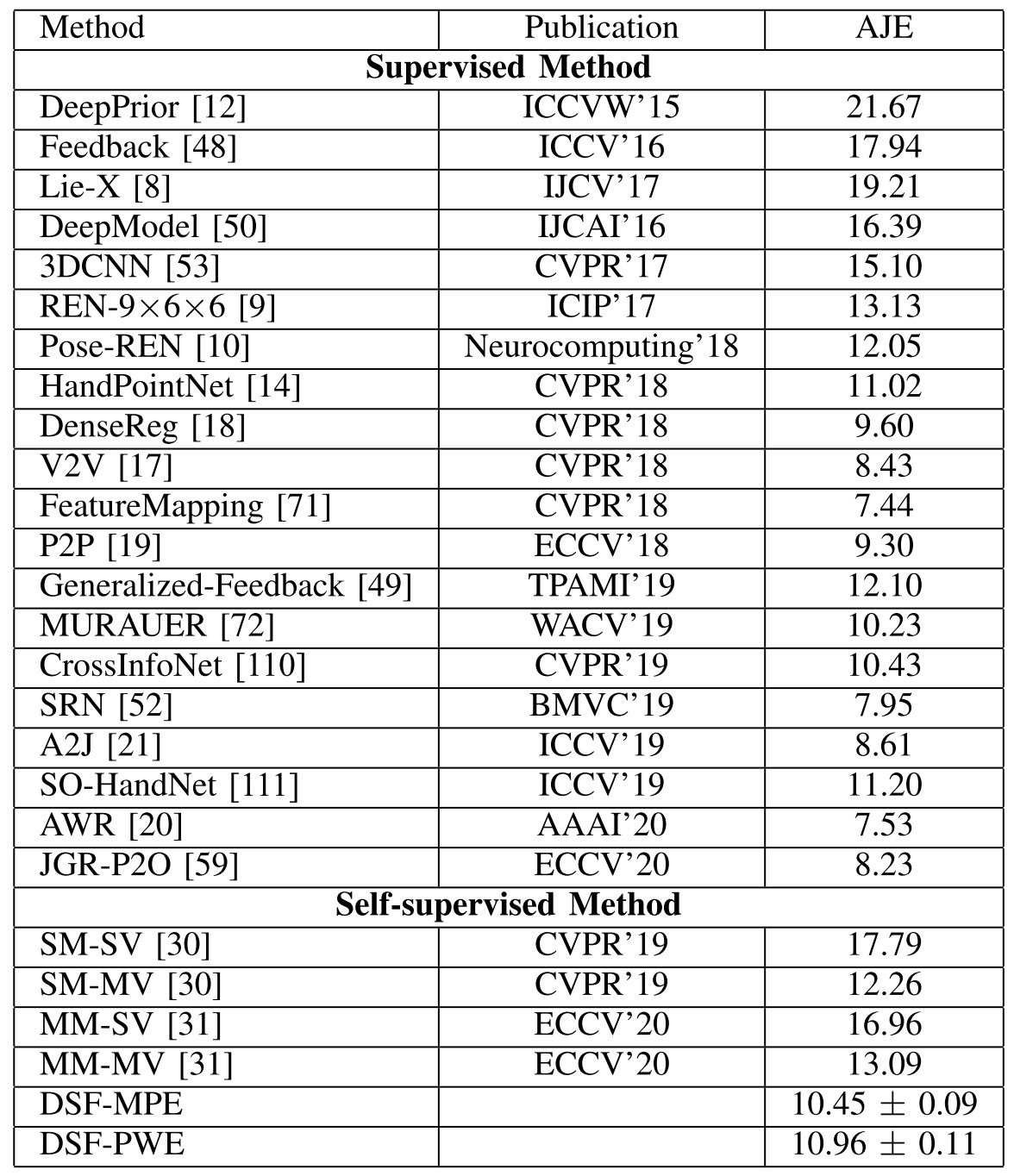
Downstream task: Skeleton-based Action Recognition
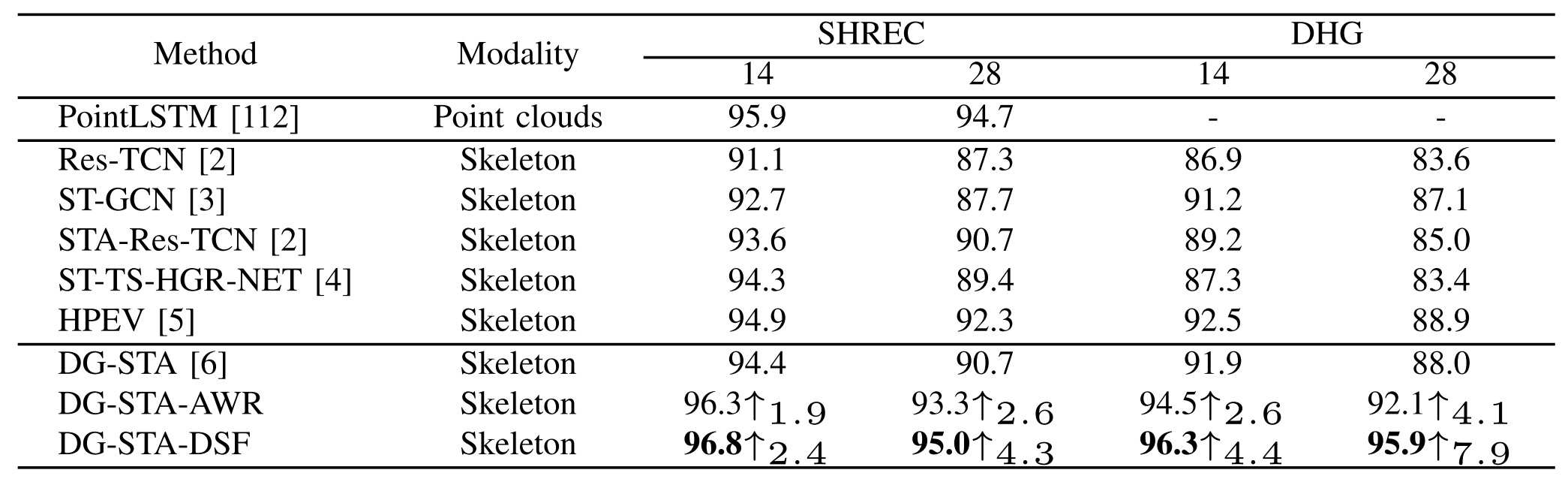
Using the 3D skeleton generated by DSF can greatly improve the accuracy of the skeleton-based action recognition, which shows that our method has strong application potential in downstream tasks.
Conclusion
In this paper, we propose a Dual-branch Self-boosting Framework (DSF) to achieve accurate, robust and flexible 3D hand pose and model estimation without using any labeled real data.
1️⃣ Firstly, we propose an image-to-image translation technology to reduce the domain gap between synthetic data and real data, which significantly improves the effect of network pre-training.
2️⃣ Secondly, we propose a dual-branch self-boosting network that can maintain the accuracy of 3D pose estimation and the robustness and flexibility of 3D hand model estimation simultaneously.
3️⃣ Through an inter-branch loss and a part-aware model-fitting loss, we fully explore the advantages of the dual-branch structure. The two branches can promote each other continuously during the self-supervised training on unlabeled real data.
✅ Our method achieves comparable results to state-of-the-art fully supervised methods and shows better generalization performance.
✅ Our method outperforms previous self-supervised methods without using paired multi-view images.
✅ In addition, our method greatly improves the accuracy of skeleton-based gesture recognition, which shows that our method has strong application potential in downstream tasks.
Supplementary Videos
This video shows the performance of our method on the test set of the NYU dataset.
This video shows the performance of our method on the test set of the ICVL dataset, qualitatively demonstrating that our method can generate more reasonable hand poses than annotations. We use red circles to locate errors in annotations.
This video shows the performance of our method on the MSRA dataset (subject 0), qualitatively demonstrating that our method can generate more reasonable hand poses than annotations. We use red circles to locate errors in annotations.
Bibtex
@ARTICLE{9841448,
author={Ren, Pengfei and Sun, Haifeng and Hao, Jiachang and Qi, Qi and Wang, Jingyu and Liao, Jianxin},
journal={IEEE Transactions on Image Processing},
title={A Dual-Branch Self-Boosting Framework for Self-Supervised 3D Hand Pose Estimation},
year={2022},
volume={31},
number={},
pages={5052-5066},
doi={10.1109/TIP.2022.3192708}}